Global Models
The diversity of the PARIS REINFORCE project’s entire modelling ensemble is an asset and, in order to make efficient use of the available models, we must inform on their potential uses for climate policy support. Evidently, not all questions can be equally addressed by all models, nor will all models that can address a specific question give similar answers. The policy issues to be addressed by the models are mainly related to mitigation of and adaptation to climate change, although all eight models are better suited for studying mitigation options than they are for delving into adaptation; as well as to overall sustainable development.
This section begins with the presentation of the main drivers, or exogenous variables, such as socioeconomic assumptions, that are considered essential inputs for the modelling simulations. Once defined, the mechanisms involved in each model in the climate action scenarios are defined. After considering these drivers and mechanisms, we take stock of policy instruments that can be implemented in each model either directly or after specific modelling adjustments. Finally, we provide a short overview of how a transition pathway is calculated as well as of example use cases for each model. A detailed account of the information included in this section is presented in the documentation of Section 3.
Before exploring the capabilities of the eight models, we first present here the main drivers and exogenous assumptions necessary for the modelling simulations. This includes GDP and population growth on the one hand, which are the core socioeconomic pillars of integrated assessment modelling input and usually based on universal socioeconomic databases like the Shared Socioeconomic Pathways (SSP) framework (Riahi et al., 2017), and other key parameters on the other, which largely vary among the models.
Regarding the former, all eight models require inputs on population projections. This is the case for GDP growth, with the exception of the general equilibrium models (DICE, as an optimal growth model, included), which essentially require GDP data for calibration but then endogenously calculate GDP along with other macroeconomic indicators, such as consumption, trade, employment, investments and public finance indices.
As far as other key parameters are concerned, these vary greatly by model, mainly due to their mathematical and economic structure. For example, computable general equilibrium models like GEMINI-E3 and ICES require detailed information on national and international social accounting matrices, government transactions and other flows, which are used for calibrating the models and usually draw from specific databases. But what all models have in common, beside specific socioeconomic assumptions, are data requirements for technological specifications, in energy markets, electricity generation mixes and, where applicable, vehicle fleet size and structure. Main socioeconomic assumptions and key parameters for all eight models are presented in the table below.
Model | Population Growth | Economic Growth | Other Key Parameters |
---|---|---|---|
GCAM | Exogenous | Exogenous | Labour participation and productivity; energy technology costs, performance, water requirements; agricultural technology crop yields, costs, carbon contents, water requirements, fertiliser requirements; resources (fossil fuels, wind, solar, uranium and groundwater); and policies (emissions constraints, renewable portfolio standards, etc.). |
TIAM | Exogenous | Exogenous | Fossil fuel availability and cost (i.e. supply curves); technology availability and costs; information about under construction/planned/possible energy technologies; regional emissions reduction goals; and energy efficiency improvements rates. |
MUSE | Exogenous | Exogenous | Degree to which energy demand and demand for other goods and services resulting in GHG emissions change over the time horizon (future demand projections of each service in each region are based on population and GDP growth). |
42 | Exogenous | Exogenous | Vehicle fleet and structure; structure of electricity and heat production; sectoral energy intensities; fuel efficiency for cars and trucks; and fuel efficiency for electricity and heat plants. |
GEMINI-E3 | Exogenous | Endogenous (exogenous for calibration) | Energy markets in physical units; detailed social accounting matrices; indirect taxation; and government expenditures (for calibration). |
ICES | Exogenous | Endogenous (exogenous for calibration) | National social accounting matrices; economic flows related to fuel-specific energy production and consumption; international sectoral statistics; international transactions among governments and transactions/flows between government and private households, and public debt (for calibration). |
DICE | Exogenous | Endogenous | Overall savings rate for physical capital and rate of control of GHG emissions. |
E3ME | Exogenous | Endogenous (exogenous for calibration) |
This section presents the different mitigation and adaptation options included in the eight models, as detailed in the following tables.
Upstream technologies like hydrogen production and synthetic fuel production are covered in detail as mitigation options in MUSE, which covers almost all existing technologies. GCAM and TIAM only include coal and biomass to liquids production, with and without carbon capture and storage/sequestration (CCS); but, like MUSE, both models include most hydrogen production technologies. GCAM however additionally features thermal splitting but not biomass to hydrogen (with CCS). It should be noted that E3ME also includes a limited number of upstream technologies but not to the same detail as the other three models.
With the exception of DICE, all models cover a large set of mitigation options in the electricity generation sectors, ranging from nuclear to renewables: CCS, hydro, solar photovoltaic (PV) and concentrating solar power (CSP), onshore and offshore wind turbines, biomass (with and without CCS) and geothermal; the general equilibrium models (GEMINI-E3 and ICES), however, feature less technological detail, while contrary to most other models 42 also includes nuclear fusion (along with TIAM) and do not distinguish onshore and offshore wind. Regarding heat generation, only 42, MUSE and TIAM feature significant detail, while GCAM includes heat from biomass.
In the building sector, the energy system (42 and MUSE) and partial equilibrium (GCAM and TIAM) models include mitigation options to lesser or larger extent; while GEMINI-E3 includes building technologies as an aggregated parameter; and ICES only covers behavioural changes and electricity for cooling as exogenous shifts in households’ energy demand.
In the transport sector, GCAM, TIAM, MUSE and 42 again cover all or almost all technologies for road transportation, while GEMINI-E3 only includes fully electric vehicles. The technological options for GHG emission reductions in aviation and shipping are relatively limited, and only in the four abovementioned models, covering biofuels (GCAM, TIAM, MUSE, 42), hydrogen (TIAM), electricity (MUSE) and efficiency (TIAM, 42) in aviation; and gas (MUSE, TIAM, 42), hydrogen (TIAM, MUSE), biofuel (all four) and efficiency (42) in shipping. Railways electrification is also available in all four models (and GEMINI-E3), while MUSE also includes hydrogen fuel cell rail. Finally, modal shift can be used to favour low-carbon transports in GCAM and GEMINI-E3, which two along with ICES also include behavioural changes in the transport sector.
For the manufacturing sectors, GCAM, TIAM, MUSE and 42 include various mitigation options for heat processing, machine drives, steam, combined heat and power (CHP); while GEMINI-E3 covers some of them as an aggregate. In addition, MUSE also includes CCS options in industry, as is the case for TIAM as well, which however also includes direct air capture (DAC).
To mitigate GHG emissions from agriculture, MUSE, 42, TIAM and GEMINI-E3 cover energy use in detail, whereas GCAM includes mitigation options for land yield maximisation and improved feeding. GCAM, GEMINI-E3 and ICES also cover behavioural change mitigation options, such as reductions in demand.
In land use, land-use change and forestry, the available mitigation options in the modelling ensemble are afforestation (GCAM, TIAM and MUSE), land protection (GCAM and MUSE) and biomaterials (GCAM).
The models documented in this deliverable do not in general produce outputs that are directly relevant to adaptation considerations but can offer indirect insights to inform adaptation planning. The GCAM model has some consideration of adaptation, directly allowing the set-aside of protected land, as well as directly calculating the additional cooling requirement of buildings as the climate warms, while ICES covers restrictions to water use.
In principle, adaptation measures could be included as a consideration in all models’ simulations of mitigation pathways, through for example limiting bioenergy resources (e.g. to represent adaptation to crop yield reductions in a warming climate), or increasing building cooling requirements exogenously given the expectation of a warmer climate. However, these models do not project the impacts of a changing climate, so they are of limited use for adaptation considerations.
GCAM | TIAM | MUSE | 42 | GEMINI-E3 | ICES | DICE | E3ME | ||||
---|---|---|---|---|---|---|---|---|---|---|---|
Mitigation Measures | Upstream Technologies | Synthetic fuel production | x | x | x | x | |||||
Hydrogen production | x | x | x | x | |||||||
Electricity and Heat Generation Technologies | Electricity generation | CCS | x | x | x | x | x | x | |||
Nuclear fission | x | x | x | x | x | x | |||||
Nuclear fusion | x | x | |||||||||
Hydro | x | x | x | x | x | x | x | ||||
Biomass | x | x | x | x | x | ||||||
Biomass with CCS | x | x | x | x | |||||||
Geothermal | x | x | x | x | x | ||||||
Solar PV & CSP | x | x | x | x | x | x | x | ||||
Wind onshore & offshore | x | x | x | x | x | x | x | ||||
Heat generation | CCS | x | x | ||||||||
Geothermal | x | x | |||||||||
Biomass | x | x | x | x | |||||||
Buildings | Heating | Gas replacing oil / coal | x | x | x | x | x* | ||||
Biofuels | x | x | x | x | |||||||
Electricity | x | x | x | x | x* | ||||||
Hydrogen | x | x | x | ||||||||
Solar thermal | x | x | x | ||||||||
Building shell efficiency | x | x* | |||||||||
Lighting | Efficient lighting | x | x | x* | |||||||
Appliances | Efficient appliances | x | x | x* | |||||||
Cooling | Electricity | x | x | x | x | ||||||
Behaviour change (less energy service demand) | x | x |
*: Aggregated parameters for GEMINI-E3 model
GCAM | TIAM | MUSE | 42 | GEMINI-E3 | ICES | DICE | E3ME | ||||
---|---|---|---|---|---|---|---|---|---|---|---|
Mitigation Measures | Transport | Road | Gas (LNG / CNG) vehicles | x | x | x | x | ||||
Hybrid electric vehicles | x | x | x | x | |||||||
Fully electric vehicles | x | x | x | x | x | ||||||
Hydrogen fuel cell vehicles | x | x | x | ||||||||
Biofuels in fuel mix | x | x | x | x | |||||||
Efficiency | x | x | |||||||||
Rail | Electric rail | x | x | x | x | x | |||||
Hydrogen fuel cell rail | x | ||||||||||
Efficiency | x | x | x | ||||||||
Aviation | Biofuels in fuel mix | x | x | x | x | ||||||
Hydrogen planes | x | ||||||||||
Electric planes | x | ||||||||||
Efficiency | x | x | |||||||||
Shipping | Gas (LNG / CNG) | x | x | ||||||||
Hydrogen | x | x | |||||||||
Biofuels in fuel mix | x | x | x | x | |||||||
Efficiency | x | ||||||||||
Modal shifts | x | x | |||||||||
Other behaviour changes (e.g. travelling less) | x | x | x |
GCAM | TIAM | MUSE | 42 | GEMINI-E3 | ICES | DICE | E3ME | ||||
---|---|---|---|---|---|---|---|---|---|---|---|
Mitigation Measures | Industry | Process heat | Gas replacing oil / coal | x | x | x | x | x* | |||
Biomass | x | x | x | x | |||||||
Hydrogen | x | x | x | ||||||||
Electricity | x | x | x | x | x* | ||||||
Machine drives | Gas replacing oil / coal | x | x | x | x* | ||||||
Electricity | x | x | x | x* | |||||||
Steam | Gas replacing oil / coal | x | x | x* | |||||||
Electricity | x | x | x* | ||||||||
CHP | Gas replacing oil / coal | x | x | x | x* | ||||||
Biomass | x | x | x | x | x* | ||||||
Overall industry | CCS | x | x | ||||||||
Behaviour changes (lower mat. consumption) | x | x | |||||||||
Agriculture & LULUCF | Energy use | Gas replacing oil / coal | x | x | x | x | |||||
Biomass | x | x | x | ||||||||
Electricity | x | x | x | x | |||||||
Land practices | Land yield maximisation | x | |||||||||
Animal husbandry | Improved feeding | x | |||||||||
Behaviour changes (less product demand) | x | x | x | ||||||||
Afforestation, land protection, biomaterials | x | x | x |
*: Aggregated parameters for GEMINI-E3 model
GCAM | TIAM | MUSE | 42 | GEMINI-E3 | ICES | DICE | E3ME | |||
---|---|---|---|---|---|---|---|---|---|---|
Adaptation Measures | Land | Water use restrictions | x | |||||||
Land use adaptation/planning | x | |||||||||
Urban | Additional cooling of buildings | x |
All models (can) include some form of emissions mitigation policy instruments, like taxation, emissions target quotas, standards, financial support and global temperature or radiative forcing targets; as well as, with the exception of DICE, some form of energy policy instruments, including taxes and subsidies, energy mix and efficiency targets and specific regulations (e.g. building codes, vehicle technology bans, etc.).
Land policy instruments, on the other hand, such as land protection, production quotas and land-use change emissions taxation are only included in the GCAM model, while TIAM, ICES and DICE can be modified to include some of these instruments. Afforestation targets, as a policy option, are only feasible with minor modifications to some of the models (GCAM, TIAM and DICE).
Finally, carbon border taxes (on imports) and subsidies (on exports) are only included in GEMINI-E3, ICES and (potentially) in TIAM and E3ME; while TIAM and E3ME can also be modified to include some regulation policies (like certifications).
The table below summarises policy coverage in the eight documented models.
GCAM | TIAM | MUSE | 42 | GEMINI-E3 | ICES | DICE | E3ME | ||
---|---|---|---|---|---|---|---|---|---|
Emissions mitigation policy instruments | Tax | x | x | x | x | x | (x) | x | |
Emissions target / quota (annual) | x | x | x | x | x | x | x | (x) | |
Emissions target / quota (cumulative) | x | (x) | x | (x) | x | x | (x) | ||
Regulations (emissions standards, etc…) | (x) | x | x | (x) | |||||
Financial supports (e.g. negative emissions, Green Climate Fund) | x | (x) | (x) | (x) | |||||
Global temperature/radiative forcing target | x | x | (x) | (x) | x | ||||
Energy policies instruments | Tax | x | x | x | x | x | x | ||
Subsidy | x | x | x | x | x | x | |||
Energy mix target | x | x | x | (x) | x | (x) | |||
Efficiency target | (x) | x | x | (x) | x | (x) | |||
Regulations (thermal regulation in buildings, bans on diesel cars, etc.) | x | x | (x) | ||||||
Land policies instruments | Protected lands | x | |||||||
Production quotas | x | (x) | (x) | ||||||
Carbon sink pricing / Land use change emissions tax | x | (x) | |||||||
Afforestation targets | (x) | (x) | (x) | ||||||
Trade policies instruments | Carbon border tax on imports | (x) | x | x | (x) | ||||
Carbon border supports on exports | (x) | x | x | (x) | |||||
Regulation policies (certifications, best-available technologies, etc.) | (x) | (x) |
The following table details sixteen of the seventeen SDGs set by the United Nations in 2015 for the year 2030. SDG17 on revitalising global partnership for sustainable development is excluded, as out of the scope of the featured modelling tools. Among these SDGs and with the exception of ICES which can provide insights into all SDGs, the other seven models can deliver indicators to track directly or indirectly eleven of the SDGs.
All of the models are able to report on emissions and therefore on climate action (SDG13). All models can also offer direct insights into affordable and clean energy (SDG7), with the exception of DICE: this entails analyses of renewable energy (all models), access to electricity and primary energy intensity (ICES), traditional biomass use (42 and GCAM), and a full assessment of energy commodities (TIAM, E3ME and MUSE). The same can be said for industry, innovation and infrastructure (SDG9), with the exception of GCAM and 42 that do not feature direct implications. Decent work and economic growth (SDG8) is also covered by five models, mainly via GDP (per capita) growth and employment impacts. SDG2 (zero hunger) is also included in half of the models, be looking into food prices (GCAM, GEMINI-E3 and E3ME) and overall undernourishment (ICES).
Other covered SDGs include SDG1 on poverty prevalence (ICES); SDG3 on health through physical density and life expectancy (ICES) and pollution levels linked to mortality (GCAM, TIAM and E3ME); SDG4 on literacy rate (ICES); SDG5 on gender inequality based on income distribution (E3ME); SDG6 on water and sanitation via freshwater withdrawals (ICES) and groundwater depletion (GCAM); SDG10 on inequalities (E3ME and ICES); SDG11 on sustainable cities (ICES); SDG12 on responsible production and consumption through material productivity (ICES), and footprint impacts (GCAM); SDG15 on life on forest land (ICES and GCAM) and land use change (GCAM); and SDG16 on peace, justice and institution (ICES).
Regarding ICES, a dedicated module aims at offering a comprehensive assessment of future sustainability up to 2030 (with the capacity to extend the analysis to 2050) based upon 27 indicators related to the seventeen SDGs, under different socioeconomic and policy scenarios, by combining the ICES modelling framework with a regression approach (based on historical data) to offer an internally-consistent set-up for analysing future patterns of sustainability indicators and their inter-linkages.
Measure | GCAM | TIAM | MUSE | 42 | GEMINI-E3 | ICES | DICE | E3ME |
---|---|---|---|---|---|---|---|---|
§1. No Poverty | x | |||||||
§2. Zero hunger | x | x | x | x | ||||
§3. Health | x | x | x | x | ||||
§4. Quality education | x | |||||||
§5. Gender equality | x | |||||||
§6. Clean water and sanitation | x | x | ||||||
§7. Affordable and clean energy | x | x | x | x | x | x | x | |
§8. Decent work & economic growth | x | x | x | x | x | |||
§9. Industry, innovation & infrastructure | x | x | x | x | x | x | ||
§10: Reduced inequalities | x | x | ||||||
§11: Sustainable Cities & Communities | x | |||||||
§12: Responsible production & consumption | x | x | ||||||
§13: Climate action | x | x | x | x | x | x | x | x |
§14: Life below water | ||||||||
§15: Life on land | x | x | ||||||
§16: Peace, Justice and institutions | x |
Since all eight models are significantly different, they do not operate homogeneously to calculate climate change mitigation pathways. However, some of them feature specific similarities; therefore, the models can be grouped into categories and described by the approach of each category. The models can be sorted into five groups (Nikas et al., 2019):
GCAM and TIAM are partial equilibrium models, in that they achieve an equilibrium between the supply and demand for energy in each sector represented, taking into account the changes in energy prices that result from the changes in fuels and technologies used to satisfy energy service demands in these sectors. They are not “full” equilibrium models as they do not consider the subsequent changes in the production and supply costs and demands of all services and goods in the economy—only the energy sectors. TIAM operates on a “perfect foresight” cost-optimisation basis, whereby all consequences of technology deployments, fuel extraction and energy price changes over the entire time horizon are considered when minimising the cost of the energy system, so as to provide energy service demands within specified emissions constraints. By contrast, GCAM operates on a “recursive dynamic” cost-optimisation basis, which means that, rather than considering all future time periods, it solves for the least-cost energy system in a given period, before moving to the next time period and performing the same exercise.
MUSE and 42 are energy system models, and can therefore be considered as a subcategory of the partial equilibrium modelling group, providing a detailed account of the energy sector, i.e. energy technologies and their associated costs, in order to determine the least-cost ways of attaining GHG emission reductions or the costs of alternative climate policies. They both are bottom-up models that assume short-term microeconomic equilibrium on the energy system, which is achieved by iterating market clearance across all of the sector modules, interchanging price and quantity of each energy commodity in each region. MUSE, in addition, is also an agent-based model, as it tries to determine a mitigation pathway by providing an as realistic as possible description of the investment and operational decision making in each geographical region within a sector.
GEMINI-E3 and ICES are computable general equilibrium (CGE) models, and therefore have a more detailed, multiple-sector representation of the economy and, rather than seeking optimal policies, they consider the impacts of specific policies on economic, social and environmental parameters. Their operation is similar to that of GCAM and TIAM, but differs in that market equilibrium is assumed to take place in the entire economy. Their richer representation of the economy comes at a cost in that the growth of the economy is harder to model and its structure more complex; as such, they require calibration to data on national and international socio-accounting information, as well as input in the form of a series of elasticities of substitution. Contrary to all other models, they also calculate economic indices endogenously.
Although DICE can also be considered as part of the CGE family, it is distinguished as an optimal growth, or welfare optimisation, or neoclassical model, which does not feature the same level of sectoral or geographic detail (it exclusively covers the entire global economy). It determines the climate policy and investment levels that maximise welfare (future against present consumption) over time, by identifying the emission abatement levels for each time step; its social welfare function represents the world’s well-defined set of preferences and accordingly ranks different consumption paths, with welfare increasing in per capita consumption for each generation but with diminishing marginal utility of consumption (the wealthier the world is, the less valuable an additional unit of consumption is).
Finally, E3ME is a macroeconometric model. Quite like CGE models, it is quite detailed in terms of energy technologies and geographic scope, but differs in that it does not assume that consumers and producers behave optimally or that markets clear and reach equilibrium in the short term. Instead, it uses historical data and econometrically estimated parameters and relations to dynamically and more realistically simulate the behaviour of the economy, by assuming that markets achieve equilibrium in the longer run.
Model | Example Study | Research Question/Focus | Selected Key Findings |
---|---|---|---|
GCAM | Van de Ven et al. (2019) | Integrated policy assessment and optimisation over multiple sustainable development goals in Eastern Africa | The analysis shows that support for biogas technology should be prioritised in both the short and long term, while financing liquefied petroleum gas and ethanol technologies also has synergetic climate, health and energy access benefits. Instead, financing PV technologies is mostly relevant for improving energy access, while charcoal and to a lesser extend fuelwood technologies are relevant for curbing GHG emissions if their finance is linked to land policies. |
TIAM | Realmonte et al. (2019) | An investigation of the role of Direct Air Capture (DAC) in meeting global 1.5oC and 2oC pathways | DAC can reduce the policy costs of mitigation in the near-term, but there are many uncertainties about its scale-up potential and, if we ease mitigation efforts and rely on DAC, even if it does not deliver large-scale removals, we will overshoot the Paris climate goals. |
MUSE | Kerdan et al. (2019a) | Gas infrastructure pathways for the southern states of Brazil | Results suggest that, due to the expected increase in regional gas demand in South Brazil, the existing gas infrastructure would require additional investments. Depending on the renegotiation outcomes between Brazil and Bolivia (i.e. either maintaining constant, halving, or halting the Bolivian import of gas), natural gas demand could be covered by a share of alternative supply options, such as an increase in pre-salt production, LNG imports and imports from a new Argentinian pipeline. |
42 | Ivanter et al. (2018) | How to Boost the Development of the Russian Economy: Priority Actions | A set of priority directions of the economic policy, primarily in investment activity, development of the domestic market, as well as financial and organisational support for the suggested actions. |
GEMINI-E3 | Babonneau et al. (2018a) | The evaluation of the Paris Intended Nationally Determined Contributions (INDCs) and the design of fair agreements concerning additional abatements up to 2050. | Results confirm the weakness of INDC pledges. Nevertheless, it shows that, with political determination, an equitable burden-sharing agreement can be achieved with very reasonable costs for all nations of approximately 0.8% of total discounted household consumption. With a more ambitious 1.5°C target, the global cost is multiplied by a factor of four revealing the stringency of such an objective. |
ICES | Campagnolo & Davide (2019) | An assessment of climate mitigation co-benefits or side-effects on poverty and inequality, in respect to the Paris Agreement. | A full implementation of the emission reduction contributions, stated in the NDCs, is projected to slow down the effort to reduce poverty by 2030 (+4.2% of the population below the poverty line compared to the baseline scenario), especially in countries that have proposed relatively more stringent mitigation targets and suffer higher policy costs. Conversely, the impact of climate policy on inequality shows opposite sign but remains very limited. If financial support for mitigation action in developing countries is provided through an international climate fund, the prevalence of poverty will be slightly reduced at the aggregate level, but the country-specific effect depends on the relative size of funds flowing to beneficiary countries and on their economic structure. |
DICE | Gramkow and Anger-Kraavi (2019)) | Exploring a transformation of Brazil’s economy, with a focus on manufacturing sectors, while contributing to the Paris targets | The correct mix of green stimulus can help modernise and decarbonise the Brazilian manufacturing sectors and the country’s economy grow faster (by up to 0.42% compared to baseline) while its CO2 emissions decline (by up to 14.5% in relation to baseline). Investment levels increase, thereby strengthening exports’ competitiveness and alleviating external constraints to long-term economic growth in net terms. Scaling up green fiscal stimulus in manufacturing sectors globally needs to be considered as one of the main policy measures helping with transformation to a low-carbon economy, especially in the developing world. |
Regarding the use of global integrated assessment models, a legitimate question has been raised, both in the literature and in the policy world, around the levels of trust that people (whether scientists, policymakers, or other stakeholders) should have in these models and their outputs (Doukas and Nikas, 2020). That is, especially, considering the underlying assumptions driving them (Kelly and Kolstad, 1999) and uncertainty ranges (Doukas et al., 2018), as well as the extent to which these are communicated alongside the results.
It is unavoidable that models, such as those documented in I2AM PARIS, cannot provide a complete representation of the world, owing to the fact that in many ways the future is unknown, and furthermore there is incomplete knowledge of past dynamics governing energy, agricultural, land and environmental systems that are represented by these models.
Despite this challenge, these models are intended to be trusted, and seen as useful and valid, by both the scientific community and—equally if not more importantly—stakeholders such as policy- and decision-makers, who will plan low-carbon strategies on their basis. Here we briefly detail the steps that have been applied in developing and using the models, as well those that will be applied in the context of the PARIS REINFORCE project, in order that such trust and validation is achieved.
The workflow is illustrated in the following figure, based on the relevant literature on evaluation and validation of integrated assessment models and drawing from the primary elements of (Schwanitz, 2013):
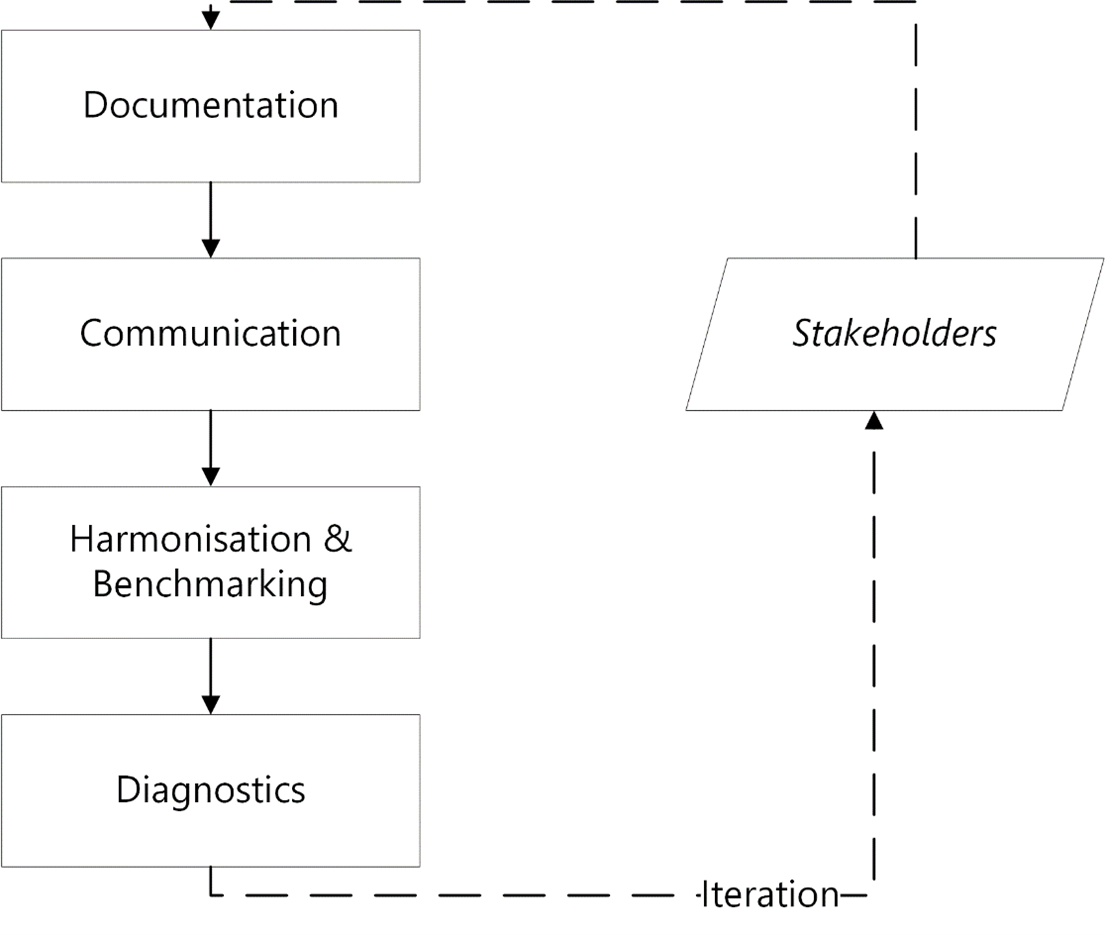
Model validation process of models in PARIS REINFORCE
In particular, very early in the project the consortium has put significant effort in documenting each of the employed models’ capabilities, in terms of geographic disaggregation, sectoral representation, types of greenhouse gas and pollutant emissions accounted for, technological detail, policy representation, socioeconomic inputs and outputs, and representation of metrics relevant to non-climate SDG indicators. It has done so for every global model in the consortium, (a) in detailed and technical format for experts’ consideration (see here), (b) in a dynamic interface and easy-to-digest language for non-experts to comprehend and map their requirements onto (see here), and (c) in a comparative setting, allowing all stakeholders to understand which models should be employed for which policy questions (as in this page). As part of this step, the project released and shared with stakeholders a policy brief on ‘what can our models do?’ (link).
Secondly, as with national/regional models for the EU and countries/regions outside the EU, the global modelling approach is in line with the “prolonged nature of model validation” (Barlas, 1996). This approach integrates opinions from the scientific community with the perspectives of stakeholders and external experts that will ultimately use the model results. PARIS REINFORCE will, in this respect, undertake active engagement with global stakeholders aimed at communicating what the models are, as well as what they can do, including a presentation of the modelling approach, preliminary results, and a discussion of the types of inputs and outputs the models produce and how they do this. This interaction will therefore provide an opportunity to place our models’ results in the context of previous results from other global low-carbon pathways modelling activities. They will also allow the project, not only to clearly communicate the modelling capabilities, features, and questions they have been asked to address in the past, but also to co-create the most pertinent questions stakeholders would like the models to address in the context of PARIS REINFORCE, in light of this well-informed stakeholder perspective. The project workflow involves first undertaking global, regionally-disaggregated modelling to explore the regional dynamics of emissions and energy/agricultural and land system transitions globally, before discussing the realism, feasibility and validity of such results with both global and regional stakeholders. In this way, global models can undertake scenarios informed by national stakeholders in various regions around the world, improving the real-world relevance of their outputs.
Third, a central aspect of achieving real-world relevance is to undertake basic harmonisation/benchmarking of the models, via targeted validity checks. This includes ensuring that base-year emissions, socio-economic assumptions, policies, and energy/agricultural/land system representations are in line with the most up-to-date verified information, and that such inputs are to the extent possible harmonised across the models used in a multi-model analysis; this is not to strip models of their invaluable diversity in the way they behave in response to specific stimuli as well as the theoretical foundations underpinning them, but quite the contrary to allow the consortium to later map the resulting ranges onto this diversity rather than uncertainties associated with ad hoc inputs assumed for each model. This also includes technology costs and performance variables. The underlying detailed protocol for achieving this has been documented in Giarola et al.
Fourth, “diagnostic” tests are and will be run for each model, to check that its responses to key input variable changes, such as stringency of climate policy (as represented by emissions targets, carbon prices, or combinations thereof), are in line with common expectations and compared to other results and models covering the same/similar regions and/or a priori defined stylised behaviours. For global models, this includes comparing the resulting ranges of the inter-comparison exercises to those of similar studies in the scientific or grey literature; for example, in building a robust reference scenario looking at current policy efforts (Sognnaes et al.), comparisons have been drawn against recent similar scientific endeavours like (Roelfsema et al., 2020) or high-impact reports like the International Energy Agency’s 2019 World Energy Outlook (IEA, 2019). These checks will build on the normal standard practice undertaken by each modelling group to regularly check the model code and ensure that errors/bugs are identified and eliminated, and to report the model’s performance alongside its results.
Finally, this evaluation process will be carried out in an iterative process throughout the project. It will be documented to ensure that models perform without unexplained dynamics in both reference and mitigation cases. A key element of this will be in taking documented modelling results back to stakeholders in the second half of the project, when they will be able to understand the behaviours of the models under increasingly stringent mitigation scenarios, and to ask why the models respond in the way that they do.
In combination, these evaluation steps cover the primary elements of the workflow suggested by Schwanitz (2013) on model validation. The models’ conceptual framework has to a large extent already been evaluated (and will be clearly communicated with stakeholders) as following from a principle of identifying least-cost pathways to low-carbon futures given the technological other input assumptions (like technology constraints, socio-economic dynamics, and representations of policies). The I2AM PARIS platform is intended to represent a major advance in communicating in a clear, accessible and attractive way the features, objectives, coverage, capabilities and limitations of models. Such documentation has been and will be accompanying our stakeholder interactions. Model structure and responses are being tested through both diagnostics as well as comparisons with other published low-carbon and reference case pathways. Whilst most modelling groups explicitly draw on a vast range of literature and comparative studies to understand the extent to which their results are similar to or different from others, and if so why, in PARIS REINFORCE we will explicitly undertake and present such comparisons throughout our modelling, to help better build trust in the results and the models themselves.
For further information on what our models can deliver click here.
- Barlas, Y. (1996). Formal aspects of model validity and validation in system dynamics. System Dynamics Review: The Journal of the System Dynamics Society, 12(3), 183-210.
- Doukas, H., & Nikas, A. (2020). Decision support models in climate policy. European Journal of Operational Research, 280(1), 1-24.
- Doukas, H., Nikas, A., González-Eguino, M., Arto, I., & Anger-Kraavi, A. (2018). From integrated to integrative: Delivering on the Paris Agreement. Sustainability, 10(7), 2299.
- Giarola, S., Mittal, S., Vielle, M., Perdana, S., Campagnolo, L., Delpiazzo, E., Bui, H., Anger-Kraavi, A., Kolpakov, A., Sognnaes, I., Peters, G.P., Hawkes, A., Koberle, A., Grant, N., Gambhir, A., Nikas, A., Doukas, H., Moreno, J., & Van de Ven, D.J. (Reviewed & Revised). Challenges in the harmonisation of global integrated assessment models: a comprehensive methodology to reduce model response heterogeneity. Science of the Total Environment.
- IEA. (2019). World Energy Outlook. IEA. Available at: https://www.iea.org/reports/world-energy-outlook-2019.
- Kelly, D. L., & Kolstad, C. D. (1999). Integrated assessment models for climate change control. International yearbook of environmental and resource economics, 2000, 171-197.
- Nikas, A., Doukas, H., & Papandreou, A. (2019). A detailed overview and consistent classification of climate-economy models. Understanding risks and uncertainties in energy and climate policy, 1-54.
- Roelfsema, M., van Soest, H. L., Harmsen, M., van Vuuren, D. P., Bertram, C., den Elzen, M., ... & Vishwanathan, S. S. (2020). Taking stock of national climate policies to evaluate implementation of the Paris Agreement. Nature Communications, 11(1), 1-12.
- Schwanitz, V. J. (2013). Evaluating integrated assessment models of global climate change. Environmental modelling & software, 50, 120-131.
- Sognnaes, I., Gambhir, A., Van de Ven, D.-J., Nikas, A., Anger-Kraavi, A., Bui, H., Campagnolo, L., Delpiazzo, E., Doukas, H., Giarola, S., Grant, N., Hawkes, A., Koberle, A., Kolpakov, A., Mittal, S., Moreno, J., Perdana, S., Rogelj, J., Vielle, M., & Peters, G.P. (in revision). A multi-model analysis of long-term emissions and warming implications of current mitigation efforts. Nature Climate Change.