National / Regional Models for Countries Outside Europe
Each of the models to be used in Work Package 6 is intended to allow the development of detailed decarbonisation pathways for major and less emitting economies of the world, encompassed by the relevant model(s). The following figure shows the different country and regional coverage encompassed by all of the models used in this work package.
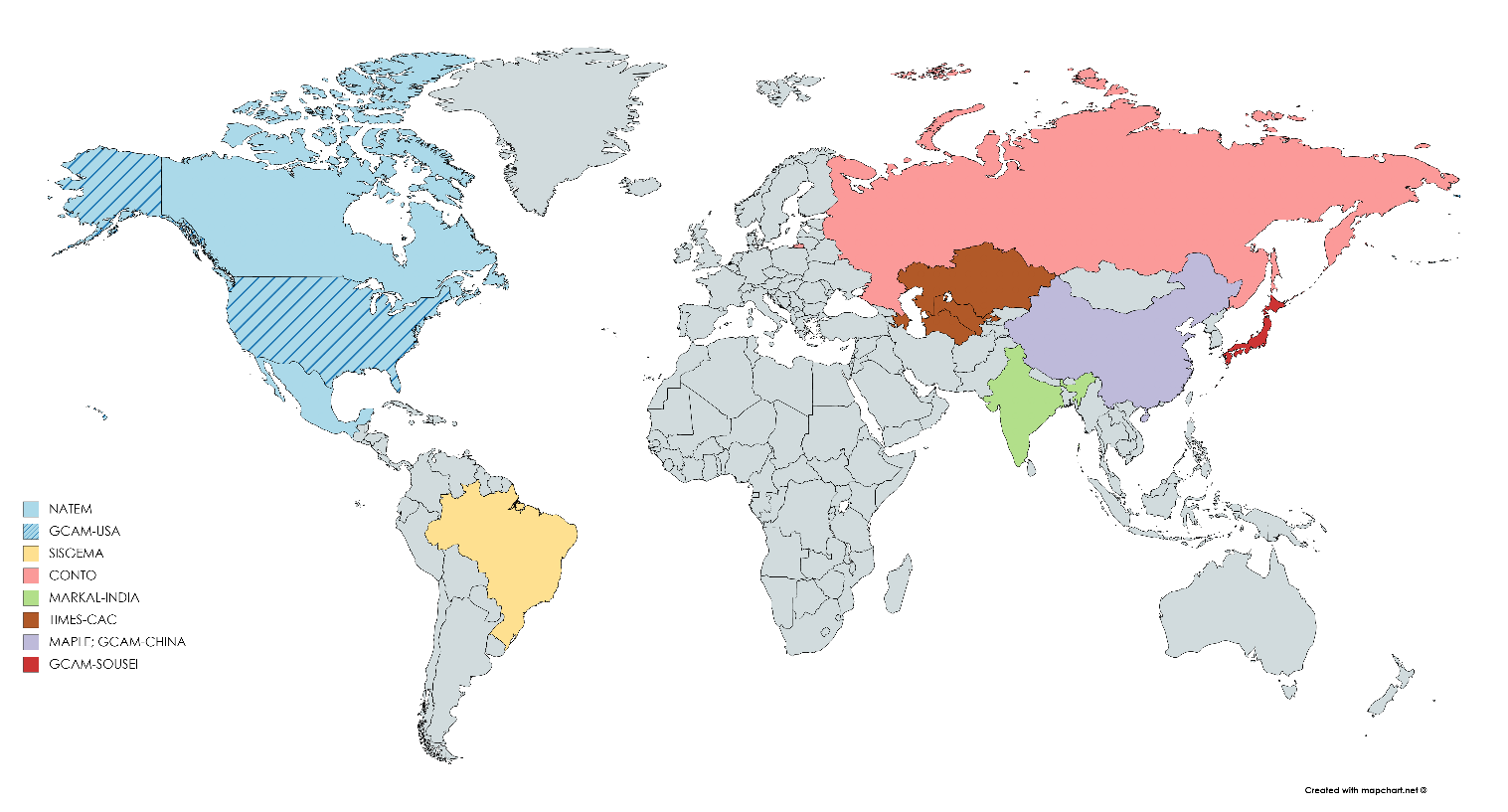
This will enable different stakeholders to provide an informed contribution into the co-design of future scenarios to develop these pathways, as well as engage with the detailed outputs that accompany each modelled pathway. The scenarios and choice mitigation pathways that result will encompass the following attributes:
- Projections for future economic and population growth that drive energy demand as well as demand for other goods and services that result in greenhouse gas (GHG) emissions (e.g. agriculture and land use)
- The technologies and measures available, by what time and to what extent, to allow mitigation
- The costs and performance characteristics of different technologies
- The emissions targets or constraints consistent with different regions’ contributions to different long-term, Paris-compliant temperature goals (i.e. well below 2oC, 1.5oC)
- The mix of policies that can be implemented to aim to achieve these emissions targets
- The interactions of different resulting mitigation pathways with other policy goals, in particular the sustainable development goals (SDGs) and adaptation goals.
The following sub-sections detail the models’ current inputs and assumptions around these factors.
Model | Population growth | Economic growth | How these drive energy and other service demand |
---|---|---|---|
CONTO | Exogenous | Endogenously calculated through previous years’ investments | Industrial and business energy demand driven by output, investment and utilisation of plant capacity. Energy demand of households and road transport driven by income and population, plus assumed energy efficiency improvements. |
GCAM-China | Exogenous | Exogenous | Industrial demand grows with GDP, with a decreasing GDP share of industrial output over time. Transport grows (at a decreasing rate) with GDP per capita, and building energy with floor space, linked to GDP per capita, with saturation levels. |
GCAM-SOUSEI | Exogenous | Exogenous | Industrial demand grows with GDP, with a decreasing GDP share of industrial output over time. Transport grows (at a decreasing rate) with GDP per capita, and building energy with floor space, linked to GDP per capita, with saturation levels. |
GCAM-USA | Exogenous | Exogenous | Industrial demand grows with GDP, with a decreasing GDP share of industrial output over time. Transport grows (at a decreasing rate) with GDP per capita, and building energy with floor space, linked to GDP per capita, with saturation levels. |
MARKAL-India | Exogenous | Exogenous | Demands for energy in households, buildings, transport and industry related to underlying GDP, sectoral and population growth drivers. |
MAPLE | Exogenous | Exogenous | Demands for energy in households, buildings, transport and industry related to underlying GDP, urbanisation and population drivers. |
NATEM | Exogenous | Exogenous | Demands for energy in households, buildings, transport and industry related to underlying GDP, sectoral and population growth drivers. |
SISGEMA | |||
TIMES-CAC | Exogenous | Exogenous | Demands for energy in households, buildings, transport and industry related to underlying GDP, sectoral and population growth drivers. |
In the case of each model, because the assumptions on population are exogenous to the model, they can be changed, to reflect underlying scenarios such as those of the Shared Socioeconomic Pathways (SSPs).
Similarly, in all models except the Russian region’s CONTO model, economic growth (either as absolute GDP growth, or GDP per capita growth, depending on the model in question) assumptions can be adjusted to reflect SSPs or alternative scenario input choices. In the case of CONTO, the GDP growth path is determined endogenously (i.e. within the model’s own calculations, as opposed to coming from an exogenous input assumption). This is done through linking investment in sectors from previous years to the growth of those sectors in future years, simulating the real-world process of economic growth. As such, different mitigation scenarios will endogenously lead to different patterns of input into, output from, and investment in different sectors of the Russian economy, thereby determining different economic growth paths.
In the case of all models, there is a functional relationship between the growth in the underlying drivers for energy demand in the transport, industrial and building sectors (such as GDP, or GDP per capita) and changes in the demand for energy services such as industrial heat, transport and building services (heating, cooling, lighting, appliances). In most cases, this relationship reflects that as the underlying driver increases, the energy demand also increases, but tends to do so at a slower—and decreasing—rate, to reflect the fact that there is a decreasing demand for additional energy services as incomes rise. In other words, the income elasticity of energy demand tends to be less than one, which means that a 1% increase in income leads to a <1% increase in a particular energy service, and furthermore this income elasticity tends to reduce over time. Some models (in particular the three regional GCAM models) explicitly represent energy service demand saturation levels in the building sector, reflecting ultimate limits to factors such as building floor space. In such cases, the income elasticity of building energy service demands falls to zero.
In addition to these assumptions on the decreasing rate of demand for energy services with increasing incomes, the CONTO model, GCAM models and MARKAL/TIMES-based models (MARKAL-India, MAPLE, NATEM and TIMES-CAC) also represent the fact that as energy prices rise (for example owing to carbon prices and/or the substitution of more expensive energy technologies for less expensive ones) the demand for energy services falls accordingly, reflecting the notion of energy price elasticity of energy demand.
The combined impact of income and price elasticity of energy demand can capture (to some extent) behavioural changes in terms of the take-up of more efficient modes of travel and changes in behaviour around the use of lighting, cooling, heating and appliances in buildings. However, more profound behavioural changes, such as large-scale shifts from private motorised transport to public transport and/or active transport (i.e. walking and cycling) are not captured in these models. As such, any scenarios that assume policies to implement and support such shifts are most likely to be implemented through exogenous input assumptions.
Finally, it should be noted that, with the exception of SISGEMA that is a land-use-only model (for Brazil), the details outlined in the table above concern only energy demand. The GCAM models also represent the evolution of demand for agricultural and land use services over time, with related greenhouse gas emissions. In these models’ cases, the demand for such services is driven by underlying socioeconomic drivers in the same way as for energy services. Further details are given in the full documentation of the GCAM model (see D7.1).
CONTO | GCAM-China | GCAM-SOUSEI | GCAM-USA | MARKAL-India | MAPLE | NATEM | SISGEMA | TIMES-CAC | |
---|---|---|---|---|---|---|---|---|---|
Upstream | Synfuels | Synfuels | Synfuels | Synfuels | Synfuels | Synfuels | |||
CtL CCS | CtL CCS | CtL CCS | CtG | BtG | CtG | ||||
BtL | BtL | BtL | BtG | BtL | CtL | ||||
BtL CCS | BtL CCS | BtL CCS | BtL CCS | ||||||
GtL CCS | |||||||||
H2 | H2 | H2 | H2 | H2 | H2 | H2 | |||
GtH CCS | GtH CCS | GtH CCS | GtH CCS | ||||||
CtH CCS | CtH CCS | CtH CCS | CtH CCS | ||||||
Electrol | Electrol | Electrol | Electrol | Electrol | Electrol | ||||
NuctH | NuctH | NuctH | |||||||
Heat | Coal CCS | Coal CCS | |||||||
Gas CCS | |||||||||
Oil CCS | |||||||||
Geo | Geo | ||||||||
Biomass | Biomass | Biomass | Biomass | Biomass | Biomass | Biomass | |||
Bio CCS | |||||||||
Electricity | Coal CCS | Coal CCS | Coal CCS | Coal CCS | Coal CCS | Coal CCS | |||
Gas CCS | Gas CCS | Gas CCS | Gas CCS | Gas CCS | Gas CCS | ||||
Nuc (fis) | Nuc (fis) | Nuc (fis) | Nuc (fis) | Nuc (fis) | Nuc (fis) | Nuc (fis) | |||
Nuc (fus) | Nuc (fus) | Nuc (fus) | |||||||
Hydro | Hydro | Hydro | Hydro | Hydro | Hydro | Hydro | Hydro | ||
Biomass | Biomass | Biomass | Biomass | Biomass | Biomass | Biomass | Biomass | ||
Bio CCS | Bio CCS | Bio CCS | Bio CCS | Bio CCS | Bio CCS | ||||
Geo | Geo | Geo | Geo | Geo | Geo | Geo | Geo | ||
Solar PV | Solar PV | Solar PV | Solar PV | Solar PV | Solar PV | Solar PV | Solar PV | ||
Solar CSP | Solar CSP | Solar CSP | Solar CSP | Solar CSP | Solar CSP | Solar CSP | |||
Wind | Wind | Wind | Wind | Wind | Wind | Wind | Wind | ||
Tidal | |||||||||
Marine |
Notes: Upstream sector technologies as follows: CtL CCS = Coal to Liquids with Carbon Capture and Storage (CCS); CtG CCS = Coal to Gas with CCS; GtL CCS = Gas to Liquids with CCS; BtG = Biomass to Gas; BtL = Biomass to Liquids; BtL CCS = Biomass to Liquids with CCS; GtH CCS = Gas to Hydrogen with CCS; CtH CCS = Coal to Hydrogen with CCS; Electrol = Electrolyis; NuctH = Nuclear to Hydrogen; Geo = Geothermal; Bio CCS = Biomass with CCS, Nuc (fis) = Nuclear fission; Nuc (fus) = Nuclear fusion; Solar CSP = Concentrating Solar Power.
Of the technologies and sectors shown in the table above, there is most variation in the different “Upstream” energy conversion processes included in the models. These are very region-specific, and include processes such as the conversion of solid fossil fuels (coal) into liquids (oil), a key strategic process for some regions which are heavily oil import-dependent. Mitigation technologies include adding carbon capture and storage (CCS) to these processes, as well as substituting biomass for coal or gas. Hydrogen is itself a zero-carbon fuel which can be used in transport and heating. But some processes to produce it (specifically, from coal and gas, when combined with water), result in CO2 emissions. Hence, low-carbon ways of producing hydrogen include the use of CCS, as well as electrolysis (provided the electricity that splits the water into hydrogen and oxygen is from low-carbon sources), and finally the high-temperature splitting of water into hydrogen and oxygen using heat from nuclear power generation.
CONTO | GCAM-China | GCAM-SOUSEI | GCAM-USA | MARKAL-India | MAPLE | NATEM | SISGEMA | TIMES-CAC | |
---|---|---|---|---|---|---|---|---|---|
Industry | Gas | Gas | Gas | Gas | Gas | Gas | Gas | Gas | |
Hydrogen | Hydrogen | Hydrogen | Hydrogen | Hydrogen | |||||
Biomass | Biomass | Biomass | Biomass | Biomass | Biomass | Biomass | Biomass | ||
Electricity | Electricity | Electricity | Electricity | Electricity | Electricity | Electricity | |||
Efficiency | |||||||||
CCS | CCS | ||||||||
Transport (road) | Gas | Gas | Gas | Gas | Gas | Gas | Gas | Gas | |
HEVs | HEVs | HEVs | HEVs | HEVs | HEVs | HEVs | |||
EVs | EVs | EVs | EVs | EVs | EVs | EVs | EVs | ||
FCVs | FCVs | FCVs | FCVs | FCVs | FCVs | FCVs | |||
Biofuels | Biofuels | Biofuels | Biofuels | Biofuels | Biofuels | ||||
Emission standards | |||||||||
Efficiency | Efficiency | Efficiency | Efficiency | Efficiency | |||||
Industry | Gas | Gas | Gas | Gas | Gas | Gas | Gas | Gas | |
Hydrogen | Hydrogen | Hydrogen | Hydrogen | Hydrogen | |||||
Biomass | Biomass | Biomass | Biomass | Biomass | Biomass | Biomass | Biomass | ||
Electricity | Electricity | Electricity | Electricity | Electricity | Electricity | Electricity | |||
Efficiency | |||||||||
CCS | CCS | ||||||||
Transport (rail) | Electricity | Electricity | Electricity | Electricity | Electricity | Electricity | Electricity | ||
Hydrogen | |||||||||
Efficiency | Efficiency | Efficiency | Efficiency | Efficiency | |||||
Transport (air) | Biofuels | Biofuels | Biofuels | Biofuels | Biofuels | Biofuels | Biofuels | ||
Efficiency | Efficiency | ||||||||
Transport (marine) | Gas | ||||||||
Hydrogen | |||||||||
Biofuels | Biofuels | Biofuels | Biofuels | Biofuels | |||||
Efficiency | Efficiency | ||||||||
Buildings (heating) | Gas | Gas | Gas | Gas | Gas | Gas | |||
Hydrogen | |||||||||
Biofuels | Biofuels | Biofuels | Biofuels | ||||||
Electricity | Electricity | Electricity | Electricity | Electricity | Electricity | Electricity | |||
Efficiency | Efficiency | Efficiency | Efficiency | ||||||
Buildings (lighting, appliances, cooling) | Efficiency | Efficiency | Efficiency | Efficiency | Efficiency | ||||
Agriculture (energy) | Biomass | ||||||||
Electricity |
Notes: EVs = Electric Vehicles; FCVs = Fuel Cell Vehicles, HEVs = Hybrid Electric Vehicles
As shown in the table above, although there variation between the demand-side mitigation technologies that can be represented in the different models, most models have a technology-rich representation of mitigation options in each sector. In addition, the models can in principle be updated so as to represent additional technologies. For example the global TIMES Integrated Assessment Model (see details in D7.1) was recently updated to represent Direct Air Capture of CO2 (DAC) . All MARKAL-TIMES models could in principle be updated in this way. Finally, a number of the models can be used to simulate behaviour changes such as demand reductions in particular sectors (e.g. transport, as a result of modal shifts, or buildings, as a result of changes to thermostat heating / cooling settings). So the previous table is primarily a description of mitigation technologies.
Measure | CONTO | GCAM-China | GCAM-SOUSEI | GCAM-USA | MARKAL-India | MAPLE | NATEM | SISGEMA | TIMES-CAC |
---|---|---|---|---|---|---|---|---|---|
Emissions targets | x | x | x | x | x | x | x | x | x |
Carbon tax | x | x | x | x | x | x | x | x | x |
Product bans / regulations | x | x | x | x | x | x | x | x | |
Energy mix targets | x | x | x | x | x | x | x | x | |
Energy efficiency targets | x | x | x | x | x | x | x | x | |
Subsidies/ finance for techs | x | x | x | x | x | x | x | x | |
Border carbon tax | x | x |
All models can include carbon pricing/taxation and CO2 emissions constraints as key policy instruments to change the pattern of energy technology take-up, thereby simulating mitigation. All models can also represent subsidies for low-carbon technologies, since each considers the costs of these technologies, as well as energy technology portfolio mix targets and energy efficiency targets.
Measure | CONTO | GCAM-China | GCAM-SOUSEI | GCAM-USA | MARKAL-India | MAPLE | NATEM | SISGEMA | TIMES-CAC |
---|---|---|---|---|---|---|---|---|---|
§1. No Poverty | x | ||||||||
§2. Zero hunger | x | x | x | ||||||
§3. Health | x | x | x | x | x | ||||
§4. Quality education | x | ||||||||
§5. Gender equality | |||||||||
§6. Clean water and sanitation | x | x | x | ||||||
§7. Affordable and clean energy | x | x | x | x | x | x | x | x | |
§8. Decent work & economic growth | x | x | x | x | |||||
§9. Industry, innovation & infrastructure | x | ||||||||
§10: Reduced inequalities | |||||||||
§11: Sustainable Cities & Communities | x | ||||||||
§12: Responsible production & consumption | x | x | x | x | |||||
§13: Climate action | |||||||||
§14: Life below water | |||||||||
§15: Life on land | x | x | x | x | x | x | |||
§16: Peace, Justice and institutions |
The table above details those SDG measures that the documented models can inform directly from their outputs. For example, all of the models track biofuels and fossil fuel use, from which estimates of particulate matter and thereby respiratory health impacts (a key metric for SDG 3) can be estimated. However, only the GCAM models and the MAPLE model actually include outputs that directly inform SDG 3. In the case of the GCAM models, the relevant metric is mortality resulting from air pollutants, whilst in the case of MAPLE it is an evaluation of health damage based on mortality, as derived from air pollutant emissions.
There are many other SDG dimensions that can be addressed by using relevant outputs from the models. For example each model produces outputs on the quantity of nuclear power deployed, which can support the investigation of non-proliferation issues relevant to the “Peace” element of SDG 16. In addition, changes in energy costs/prices as calculated by the models are not only directly relevant to SDG 7, but also indirectly to SDG 1 if interpreted as a driver of poverty.
The previous table does not indicate the degree of detail with which different SDG-relevant metrics are produced by the different models. This is because each SDG has several metrics and sub-metrics, which are influenced by a range of factors. For example, whilst many models can input into SDG 15, this is in many cases through the use of a simplified representation of afforestation and land use changes, which may mask, or at least not consider, a range of other determinants affecting the quality of life on land. A fuller description of each model’s consideration of different SDG implications is shown in the detailed documentation for each model, in Section 3.
The models listed above do not in general produce outputs that are directly relevant to adaptation considerations but can offer indirect insights to inform adaptation planning. The GCAM models have some consideration of adaptation, directly allowing the set-aside of protected land, as well as directly calculating the additional cooling requirement of buildings as the climate warms. The TIMES-CAC model allows for water use restrictions to be imposed for Kazakhstan (with the ability to replicate this for the other three countries that it represents), as well as calculating additional building cooling requirements.
In principle, adaptation measures could be included as a consideration in all models’ simulations of mitigation pathways, through for example limiting bioenergy resources (e.g. to represent adaptation to crop yield reductions in a warming climate), or increasing building cooling requirements exogenously given the expectation of a warmer climate. However, these models do not project the impacts of a changing climate, so they are of limited use for adaptation considerations.
There are three different families of energy system models in WP6:
The MARKAL-TIMES models (MAPLE, MARKAL-India, NATEM, and TIMES-CAC). These models all operate on a “perfect foresight cost-optimisation” principle, whereby the total energy system cost is minimised over the model simulation time horizon. This energy system cost consists of primary fossil fuel, biomass and uranium costs. It also includes the capital and operational costs of the energy supply technologies (e.g. power plants, heat generators, synthetic fuel production plants) and energy demand technologies (e.g. cars, light bulbs, heaters) that use these fuels (plus other, freely available energy resources such as wind, water and sunlight) either directly or after they have been converted into usable forms. This cost minimisation is calculated so as not to breach any imposed limits or constraints, such as for the annual deployment rate of specified energy technologies, or for the total emissions limit imposed. The “perfect foresight” aspect of these models means that all consequences of technology deployments, fuel extraction and energy price changes over the entire time horizon are considered in the cost-optimisation calculation.
The GCAM models (GCAM-China, GCAM-USA, and GCAM-SOUSEI). These models use a “recursive dynamic optimisation” principle. This means that the models do not consider all future time periods in the optimisation calculation. After the models solve for the least-cost energy system in a given period, they then move to the next time period and perform the same exercise. This is a marked contrast to perfect foresight optimisation models, which consider all future time periods when performing the optimisation calculation. The GCAM version used is typically operated in five-year time steps with 2100 as the final calibration year. However, the model has flexibility to be operated at a different time horizon through user-defined parameters.
• The CONTO model. In contrast to the above models, for which economic growth assumptions are exogenous, the CONTO model produces its own economic forecast, which like many other economic models, seeks to maximise economy-wide “welfare” (broadly defined as the profits of producers and the utility of consumers) and achieve an “equilibrium” between the supply of and demand for all goods and services in the Russian economy in a given time period represented. The model then moves to the next time period, representing economic growth by tracking investments and improvements in productivity over time. It tracks energy use from different fuels in the different economic sectors, as well as the household sector. It can represent mitigation of climate change (i.e. emissions reductions, through the substitution of low-carbon for high-carbon fuels) through the changing relative prices of low-carbon versus high-carbon fuels in each sector, in response for example to carbon taxes or subsidies for low-carbon fuels and technologies.
The SISGEMA model is an environmental-economic model to assess the economic and environmental impacts of alternative land uses in Brazil. The model estimates the costs of conserving and recovering forest areas, based on, respectively, land use opportunity costs and costs of fencing, inputs and labour. These cost estimates are spatially disaggregated for each of the 5,570 Brazilian municipalities. The model also estimates environmental benefits, in terms of ecosystem services provision, for each of alternative land use. SISGEMA can be integrated with Integrated assessment models (IAM)s, in such a way that it uses results from IAMs in its assumptions (such as commodities prices, land prices and carbon prices) and likewise the IAMs can update their exogenous variables (e.g. emissions pathways for the land use sector) based on results obtained from SISGEMA.
The diversity and heterogeneity of the five models allow for covering a large set of mitigation options as well as policy instruments as detailed below. These tools will be used individually in the PARIS REINFORCE project, providing a large scope of quantitative outputs. There will exist some overlapping in the results of the models allowing for an enrichment of the analysis by comparing modelling outputs. However, the five models can be complementary also due to their different focus: NEMESIS covers macroeconomic aspects as inter-sectoral economic exchange; LEAP and EU-TIMES cover the entire energy system allowing for balancing between supply and demand and a detailed analysis of technological options; FORECAST provides for in-depth analysis of mitigation options in the industrial, tertiary and residential sectors; and ALADIN completes the puzzle with a very detailed analysis of alternative road vehicles diffusion. Thus, it seems very relevant to establish a linkage process between these tools trying (i) to provide a harmonised background for all five tools, (ii) to ensure coherency in the implementation of the tools and (iii) to take advantage of the specific expertise of each tool.
At this step, no formal linkage has been established and the figure below only draws a first attempt on how this linkage could be implemented. Starting from scenario storylines and related quantitative drivers that feed all models in a harmonised manner, macroeconomic indicators (such as GDP, households’ disposable income, etc.) and sectoral economic activity will be calculated by the NEMESIS model and will be used as drivers in the others tools. In a first round, energy system models (EU-TIMES and LEAP) will determine demand, supply and particularly energy prices of which the latter will be used as input for sectoral “energy-demand” models (FORECAST and ALADIN). From this first iteration, new energy demands from industry and buildings (FORECAST) and new vehicle fleets (ALADIN) are estimated and then used in energy-system models (EU-TIMES and LEAP). When models’ outputs converge , economic variables (changes in investment requirements from energy supply and demand side, changes in energy expenditures, etc.) and new energy prices will be implemented in NEMESIS to assess the macro-sectoral impacts. A complete loop can be achieved when using new outputs of the NEMESIS model to feed the other modelling tools (ALADIN, FORECAST, EU-TIMES and LEAP).
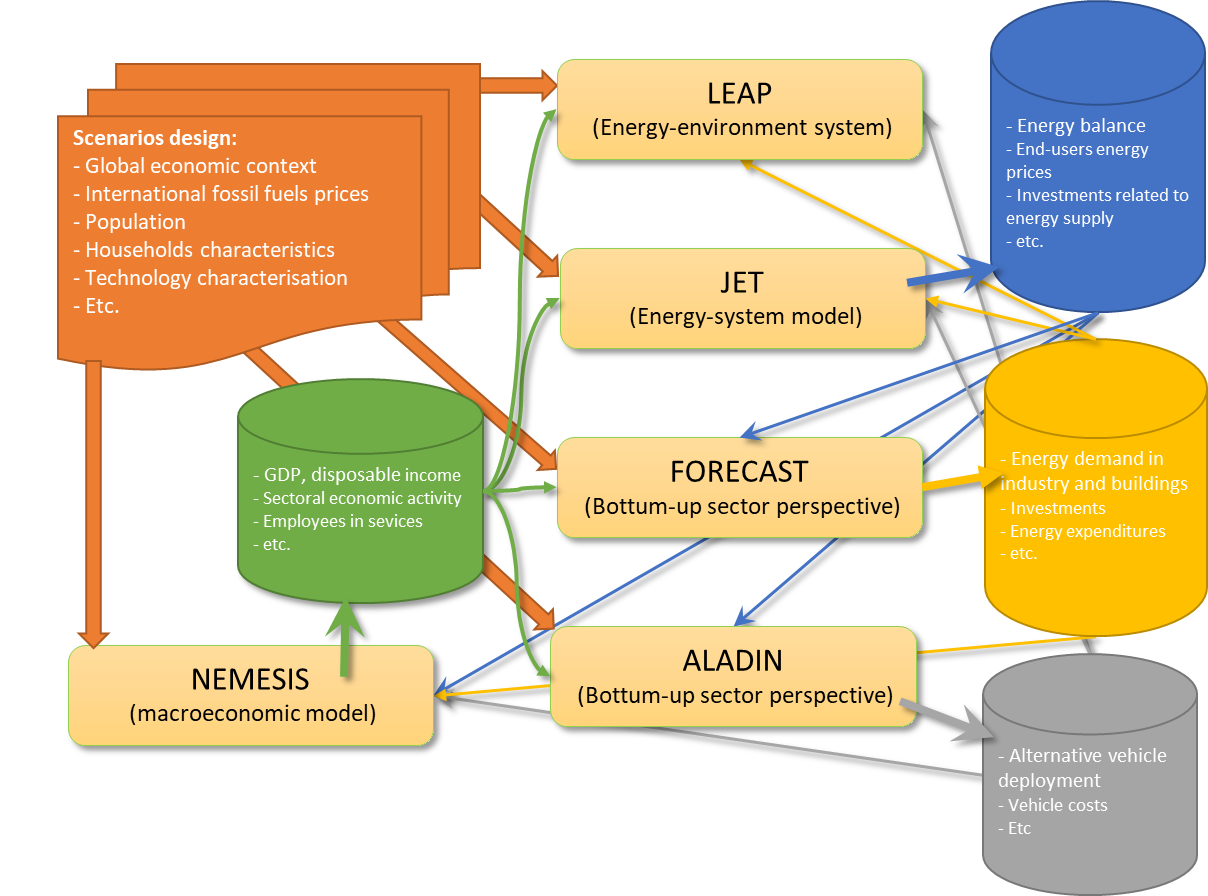
Model | Example study | Research question/focus | Selected key findings |
---|---|---|---|
CONTO | Shirov and Kolpakov (2019) | Macroeconomic impact of the energy technologies changes in Russia: Input-Output approach | Under a 1.5ºC scenario the Russian economy may lose 0.5 percent points of annual growth as a result of mitigation measures. The dependence on imports is a crucial constraint on mitigation. |
GCAM-China | Yu et al. (2019) | Carbon capture, utilisation and storage (CCUS) in China's mitigation strategy: insights from integrated assessment modelling | Across provinces and development pathways, early deployment of CCUS occurs within industrial and synthetic fuel production sectors, followed by increased deployment in the power sector by mid-century. Storage resource availability is unlikely to constrain CCUS. |
GCAM-SOUSEI | Silva Herran et al. (2019) | Global energy system transformations in mitigation scenarios considering climate uncertainties | Even when climate uncertainties are reflected at different scales across energy supply components, achieving mitigation targets needs partial decarbonisation of supply, scaling up of carbon capture and storage (CCS), and decreased energy consumption. |
GCAM-USA | Ou et al. (2018) | Environmental co-benefits of U.S. low-carbon pathways using an integrated assessment model with state-level resolution | Renewables low-carbon pathways require less water withdrawal and consumption than nuclear and carbon capture pathways, but produce higher particulate matter-related mortality costs due to use of biomass in residential heating. |
MARKAL-India | |||
MAPLE | Deep Decarbonisation Pathways Project (2019) | Key facets of decarbonisation of China in line with limiting global warming to 2ºC | Chinese CO2 emissions reduce to 4.8 Gt in 2050, 34% lower than 2010, mainly due to reduced CO2 emissions from industry and power generation. |
NATEM | Vaillancourt et al. (2018a) | The role of bioenergy in low-carbon energy transition scenarios in Quebec (Canada). | Much higher penetration of bioenergy is feasible compared to the pathway proposed by the government of Quebec in its 2030 energy policy to achieve the GHG mitigation target. |
SISGEMA | |||
TIMES-CAC | Kerimray et al. (2018). | Long-term climate change mitigation pathways in Kazakhstan in a post Paris Agreement context. | A 25% GHG emissions reduction pathway (in line with the NDC) is ambitious compared with current energy policies and mitigation actions, and would require an almost full phase-out of coal consumption in power generation by 2050. |
Regarding the use of national and regional models for countries outside Europe, like all climate-economy models, a legitimate question has been raised, both in the literature and in the policy world, around the levels of trust that people (whether scientists, policymakers, or other stakeholders) should have in these models and their outputs (Doukas and Nikas, 2020). That is, especially, considering the underlying assumptions driving them (Kelly and Kolstad, 1999) and uncertainty ranges (Doukas et al., 2018), as well as the extent to which these are communicated alongside the results.
It is unavoidable that models, such as those documented in I2AM PARIS, cannot provide a complete representation of the world, owing to the fact that in many ways the future is unknown, and furthermore there is incomplete knowledge of past dynamics governing energy, agricultural, land and environmental systems that are represented by these models.
Despite this challenge, these models are intended to be trusted, and seen as useful and valid, by both the scientific community and—equally if not more importantly—stakeholders such as policy- and decision-makers, who will plan low-carbon strategies on their basis. Here we briefly detail the steps that have been applied in developing and using the models, as well those that will be applied in the context of the PARIS REINFORCE project, in order that such trust and validation is achieved.
The workflow is illustrated in the following figure, based on the relevant literature on evaluation and validation of integrated assessment models and drawing from the primary elements of (Schwanitz, 2013):
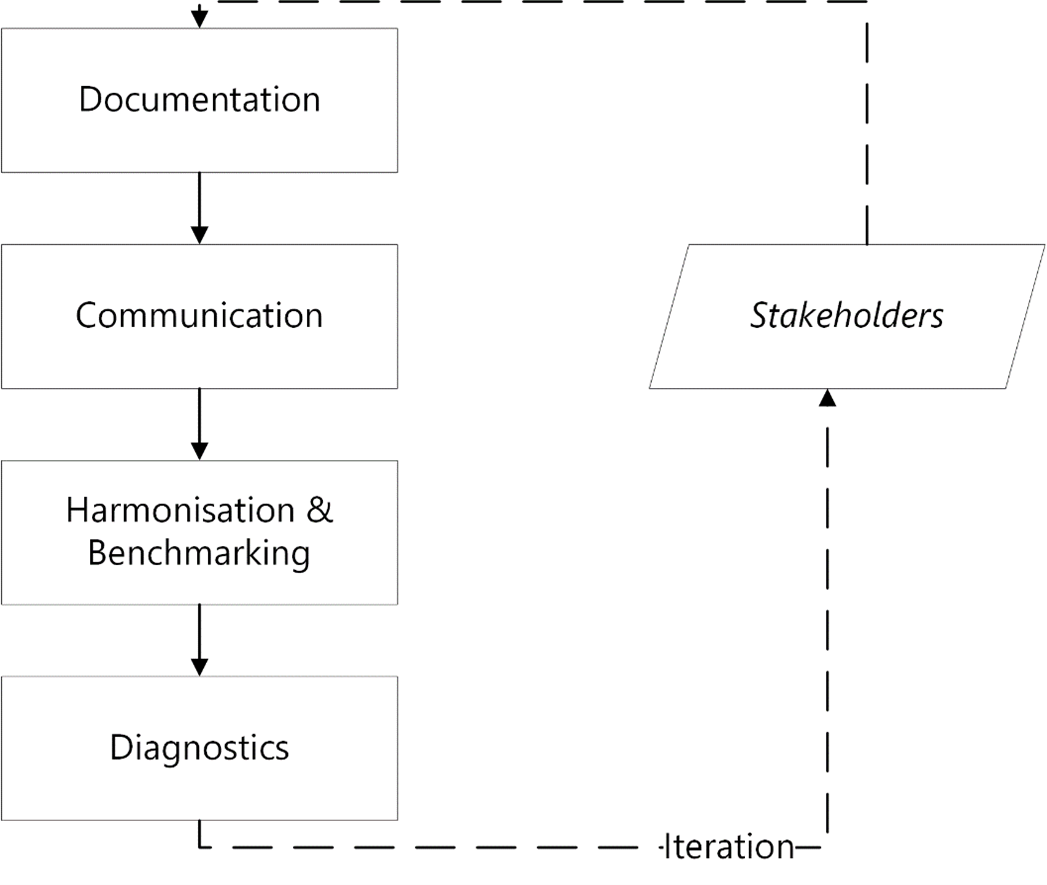
Model validation process of models in PARIS REINFORCE
In particular, very early in the project the consortium has put significant effort in documenting each of the employed models’ capabilities, in terms of geographic disaggregation, sectoral representation, types of greenhouse gas and pollutant emissions accounted for, technological detail, policy representation, socioeconomic inputs and outputs, and representation of metrics relevant to non-climate SDG indicators. It has done so for every national/regional model in the consortium, (a) in detailed and technical format for experts’ consideration (see here), ), (b) in a dynamic interface and easy-to-digest language for non-experts to comprehend and map their requirements onto (see here), and (c) in a comparative setting, allowing all stakeholders to understand which models should be employed for which policy questions (as in this page). As part of this step, the project released and shared with stakeholders a policy brief on ‘what can our models do?’ (link).
Second, as with global models and models for the EU, the non-EU regional/national modelling approach is in line with the “prolonged nature of model validation” (Barlas, 1996). This approach integrates opinions from the scientific community with the perspectives of stakeholders and external experts that will ultimately use the model results. PARIS REINFORCE will, in this respect, undertake active engagement with national stakeholders around the world aimed at communicating what the models are, as well as what they can do, including a presentation of the modelling approach, preliminary results, and a discussion of the types of inputs and outputs the models produce and how they do this. This interaction will therefore provide an opportunity to place our models’ results in the context of previous results from other national and regional low-carbon pathways modelling activities. They will also allow the project, not only to clearly communicate the modelling capabilities, features, and questions they have been asked to address in the past, but also to co-create the most pertinent questions stakeholders would like the models to address in the context of PARIS REINFORCE, in light of this well-informed stakeholder perspective. The project workflow involves first undertaking global, regionally-disaggregated modelling to explore the regional dynamics of emissions and energy/agricultural and land system transitions globally, before discussing the realism, feasibility and validity of such results with both global and regional stakeholders. In this way, global models can undertake scenarios informed by national stakeholders in various regions around the world, improving the real-world relevance of their outputs.
Third, a central aspect of achieving real-world relevance is to undertake basic harmonisation/benchmarking of the models, via targeted validity checks. This includes ensuring that base-year emissions, socio-economic assumptions, policies, and energy/agricultural/land system representations are in line with the most up-to-date verified information, and that such inputs are to the extent possible harmonised across the models used in a multi-model analysis; this is not to strip models of their invaluable diversity in the way they behave in response to specific stimuli as well as the theoretical foundations underpinning them, but quite the contrary to allow the consortium to later map the resulting ranges onto this diversity rather than uncertainties associated with ad hoc inputs assumed for each model. This also includes technology costs and performance variables. The underlying detailed protocol for achieving this has been documented in Giarola et al.
Fourth, “diagnostic” tests are and will be run for each model, to check that its responses to key input variable changes, such as stringency of climate policy (as represented by emissions targets, carbon prices, or combinations thereof), are in line with common expectations and compared to other results and models covering the same/similar regions and/or a priori defined stylised behaviours. For models covering countries and regions around the globe, this includes (a) comparing the resulting ranges of the multi-model exercises to those of similar studies looking at the regions of focus, for example (Safonov et al., 2020) for Russia, or (Wang et al., 2020) for China; and (b) comparing the resulting ranges of the multi-model exercises to those of the global model inter-comparisons carried out as part of WP7 with appropriate disaggregation at the regions of WP6 focus, e.g. (Sognnaes et al.). These checks will build on the normal standard practice undertaken by each modelling group to regularly check the model code and ensure that errors/bugs are identified and eliminated, and to report the model’s performance alongside its results.
Finally, this evaluation process will be carried out in an iterative process throughout the project. It will be documented to ensure that models perform without unexplained dynamics in both reference and mitigation cases. A key element of this will be in taking documented modelling results back to stakeholders in the second half of the project, when they will be able to understand the behaviours of the models under increasingly stringent mitigation scenarios, and to ask why the models respond in the way that they do.
In combination, these evaluation steps cover the primary elements of the workflow suggested by Schwanitz (2013) on model validation. The models’ conceptual framework has to a large extent already been evaluated (and will be clearly communicated with stakeholders) as following from a principle of identifying least-cost pathways to low-carbon futures given the technological other input assumptions (like technology constraints, socio-economic dynamics, and representations of policies). The I2AM PARIS platform is intended to represent a major advance in communicating in a clear, accessible and attractive way the features, objectives, coverage, capabilities and limitations of models. Such documentation has been and will be accompanying our stakeholder interactions. Model structure and responses are being tested through both diagnostics as well as comparisons with other published low-carbon and reference case pathways. Whilst most modelling groups explicitly draw on a vast range of literature and comparative studies to understand the extent to which their results are similar to or different from others, and if so why, in PARIS REINFORCE we will explicitly undertake and present such comparisons throughout our modelling, to help better build trust in the results and the models themselves.
For further information on what our models can deliver click here.
- Barlas, Y. (1996). Formal aspects of model validity and validation in system dynamics. System Dynamics Review: The Journal of the System Dynamics Society, 12(3), 183-210.
- Doukas, H., & Nikas, A. (2020). Decision support models in climate policy. European Journal of Operational Research, 280(1), 1-24.
- Doukas, H., Nikas, A., González-Eguino, M., Arto, I., & Anger-Kraavi, A. (2018). From integrated to integrative: Delivering on the Paris Agreement. Sustainability, 10(7), 2299.
- Giarola, S., Mittal, S., Vielle, M., Perdana, S., Campagnolo, L., Delpiazzo, E., Bui, H., Anger-Kraavi, A., Kolpakov, A., Sognnaes, I., Peters, G.P., Hawkes, A., Koberle, A., Grant, N., Gambhir, A., Nikas, A., Doukas, H., Moreno, J., & Van de Ven, D.J. (Reviewed & Revised). Challenges in the harmonisation of global integrated assessment models: a comprehensive methodology to reduce model response heterogeneity. Science of the Total Environment.
- Kelly, D. L., & Kolstad, C. D. (1999). Integrated assessment models for climate change control. International yearbook of environmental and resource economics, 2000, 171-197.
- Safonov, G., Potashnikov, V., Lugovoy, O., Safonov, M., Dorina, A., & Bolotov, A. (2020). The low carbon development options for Russia. Climatic Change, 162(4), 1929-1945.
- Schwanitz, V. J. (2013). Evaluating integrated assessment models of global climate change. Environmental modelling & software, 50, 120-131.
- Sognnaes, I., Gambhir, A., Van de Ven, D.-J., Nikas, A., Anger-Kraavi, A., Bui, H., Campagnolo, L., Delpiazzo, E., Doukas, H., Giarola, S., Grant, N., Hawkes, A., Koberle, A., Kolpakov, A., Mittal, S., Moreno, J., Perdana, S., Rogelj, J., Vielle, M., & Peters, G.P. (in revision). A multi-model analysis of long-term emissions and warming implications of current mitigation efforts. Nature Climate Change.
- Wang, H., Chen, W., Zhang, H., & Li, N. (2020). Modeling of power sector decarbonization in China: comparisons of early and delayed mitigation towards 2-degree target. Climatic Change, 162, 1843-1856.